Top-rated trending technologies, Artificial Intelligence, and Machine Learning have spiked up their significance in today’s data-drenched world. Experiencing the upward trend of growth in ever evolving landscape of technology AI and ML revolutionize the development of human society in its each and every sector.
These emerging technologies have not only improved several large-scale businesses’ capabilities but also gripped the attention of Google, Amazon, Facebook, and Microsoft. These tech giants invested heavily in research and development of Artificial Intelligence and Machine Learning and utilize them for processing and analyzing various volumes of data, driving better decision-making, generating recommendations and insights, image recognition, and many more. Used interchangeably most of the time Artificial Intelligence and Machine Learning aren’t similar in most of their aspects including scope, application, and more.it is crucial to understand the nuances that differentiate them. In this article, we will delve into the intricacies of Artificial Intelligence and Machine Learning, exploring their technological aspects and practical applications.
I. The Foundation of Artificial Intelligence:
Coined by John McCarthy in 1955, Artificial Intelligence was defined as “Science and engineering of making intelligent machines.” But now it refers to the development of computer systems that can perform tasks that typically require human intelligence. These tasks include problem-solving, speech recognition, learning, and decision-making.
As we are experiencing its involvement in even the minute aspects of human life, we can assume that it aims to remake nature by creating a society where people will co-exist harmoniously with machines. Surprisingly it’s becoming evident day by day.
B. Components of Artificial Intelligence:
Machine Learning: Machine Learning is a subset of AI that focuses on developing algorithms that allow computers to learn and improve from experience without being explicitly programmed. It involves the use of data to train models and make predictions.
Natural Language Processing (NLP): NLP enables machines to understand, interpret, and generate human-like language. This technology is the driving force behind virtual assistants and language translation services.
Computer Vision: Computer Vision enables machines to interpret and understand visual information from the world, making it possible for them to “see” and analyze images and videos.
II. Unpacking Machine Learning:
Before the advent of the golden age of Artificial Intelligence, researchers and computer scientists used to feed computers with rule-based instructions to perform a task but as soon as the demand for accuracy and automation of complex tasks further increased, a newly emerged technology Machine Learning popped out.
Categorized under the umbrella of Artificial Intelligence, Machine Learning is considered as a subset of Artificial Intelligence that focuses on teaching computers to learn from data and improve with experience. It is based on per-determined instructions being fed to computers before performing a certain task, unlike Artificial Intelligence.
- Defining Machine Learning:
Machine Learning is a subset of AI that focuses on the development of algorithms allowing computers to learn and improve from experience. It involves the use of data to train models and make predictions.
B. Types of Machine Learning:
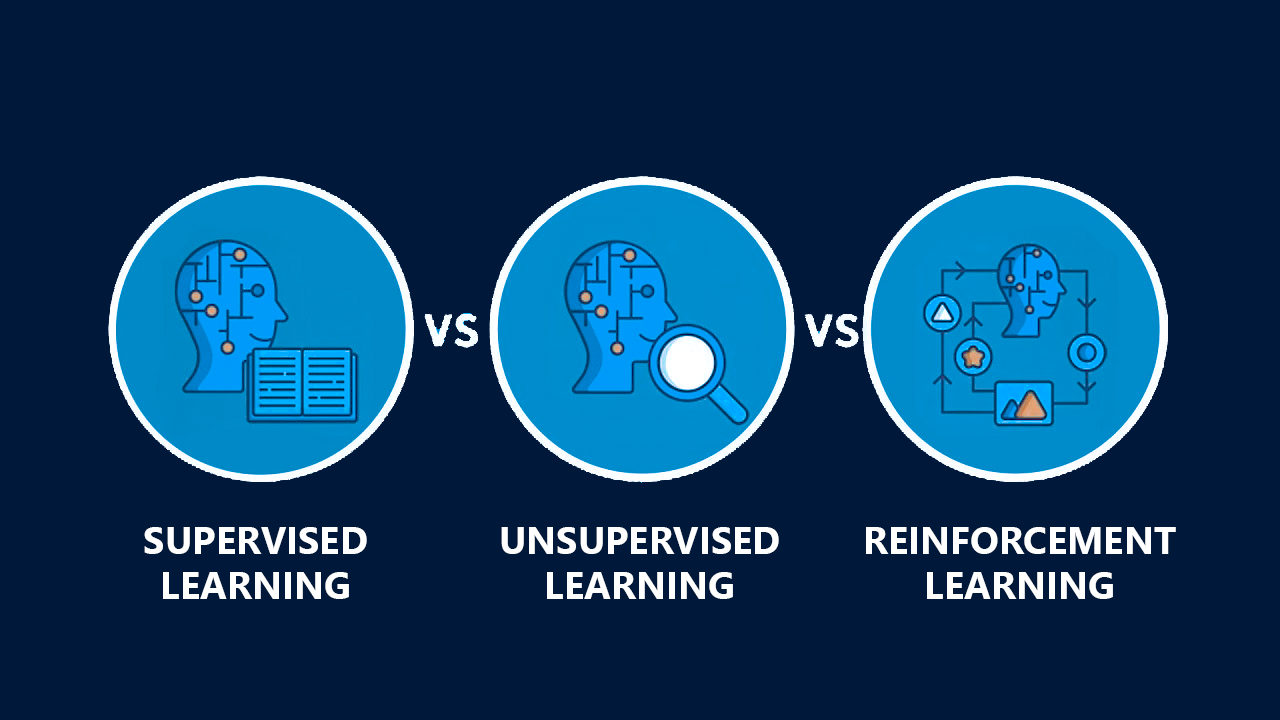
Supervised Learning:
In supervised learning, the algorithm is trained on a labeled dataset, where the input data is paired with the corresponding output. The model learns to make predictions based on this labeled data.
Unsupervised Learning:
Unsupervised learning involves training the algorithm on unlabeled data, and the model must find patterns and relationships within the data on its own. Clustering and dimensionality reduction are common applications of unsupervised learning.
Reinforcement Learning:
Reinforcement learning involves training a model to make sequences of decisions. The model receives feedback in the form of rewards or penalties based on its actions, allowing it to learn the most optimal behavior over time.
III. Practical Applications of Artificial Intelligence and Machine Learning:
A. Healthcare:
Disease Diagnosis: AI and ML are revolutionizing healthcare by aiding in disease diagnosis. For instance, machine learning algorithms can analyze medical images such as X-rays and MRIs to detect abnormalities and assist healthcare professionals in making more accurate diagnoses.
Drug Discovery: AI accelerates the drug discovery process by analyzing vast datasets to identify potential drug candidates. This not only expedites the research phase but also reduces costs associated with drug development.
B. Finance:
Fraud Detection: Machine learning algorithms are employed in the financial sector to detect fraudulent activities. These algorithms analyze transaction patterns and can identify unusual behavior, alerting financial institutions to potential fraud in real-time.
Algorithmic Trading: AI and ML play a pivotal role in algorithmic trading by analyzing market trends and making rapid decisions to optimize trading strategies. This enhances the efficiency of financial markets and allows for quicker responses to market fluctuations.
C. Marketing:
Personalized Marketing: AI enables marketers to analyze customer behavior and preferences, allowing for the creation of personalized marketing campaigns. This enhances customer engagement and increases the likelihood of conversions.
Predictive Analytics: Machine learning models can analyze historical data to predict future trends and customer behavior. This empowers businesses to make informed decisions and allocate resources effectively.
IV. Challenges and Ethical Considerations:
A. Bias in Machine Learning:
Data Bias:
Machine learning models are only as good as the data they are trained on. If the training data contains biases, the model will perpetuate those biases, leading to unfair and discriminatory outcomes.
Ethical Implications:
The use of AI and ML raises ethical concerns, particularly in areas like facial recognition, where there is a potential for misuse and invasion of privacy. Striking a balance between technological advancement and ethical considerations is crucial.
B. Lack of Explainability:
Black Box Phenomenon:
Many machine learning models operate as “black boxes,” making it challenging to understand how they arrive at specific decisions. This lack of explainability can be a barrier, especially in sensitive domains like healthcare and finance.
Interpret-able Models:
Developing interpret-able machine learning models is an ongoing challenge. Researchers and practitioners are actively working on creating models that provide transparent explanations for their decisions.
V. The Future of Artificial Intelligence and Machine Learning:
A. Advancements in Deep Learning:
Neural Networks: Deep learning, a subset of machine learning, focuses on neural networks with multiple layers. Advancements in neural network architectures, such as convolutional neural networks (CNNs) and recurrent neural networks (RNNs), continue to drive progress in various domains.
Transfer Learning:
Transfer learning allows models to leverage knowledge gained from one task to improve performance on another. This approach is particularly useful in scenarios where labeled data is scarce.
B. Integration with Edge Computing:
Edge AI:
Integrating AI capabilities at the edge, closer to where data is generated, reduces latency and enhances real-time processing. This is particularly crucial in applications like autonomous vehicles and IoT devices.
Federated Learning:
Federated learning enables model training across decentralized devices without exchanging raw data. This privacy-preserving approach is gaining traction in scenarios where data security is paramount.
Conclusion:
In conclusion, the fields of Artificial Intelligence and Machine Learning are transforming the way we interact with technology, making significant strides in various industries. Understanding the nuances of these technologies is essential for harnessing their potential while addressing challenges such as bias and lack of explainability. As we look to the future, advancements in deep learning, edge computing, and ethical considerations will shape the trajectory of AI and ML, paving the way for a more intelligent and responsible technological landscape.
Nice
this is best article . vey informative
hello nice blog